How Accurate is Agcurate and Why is it so?
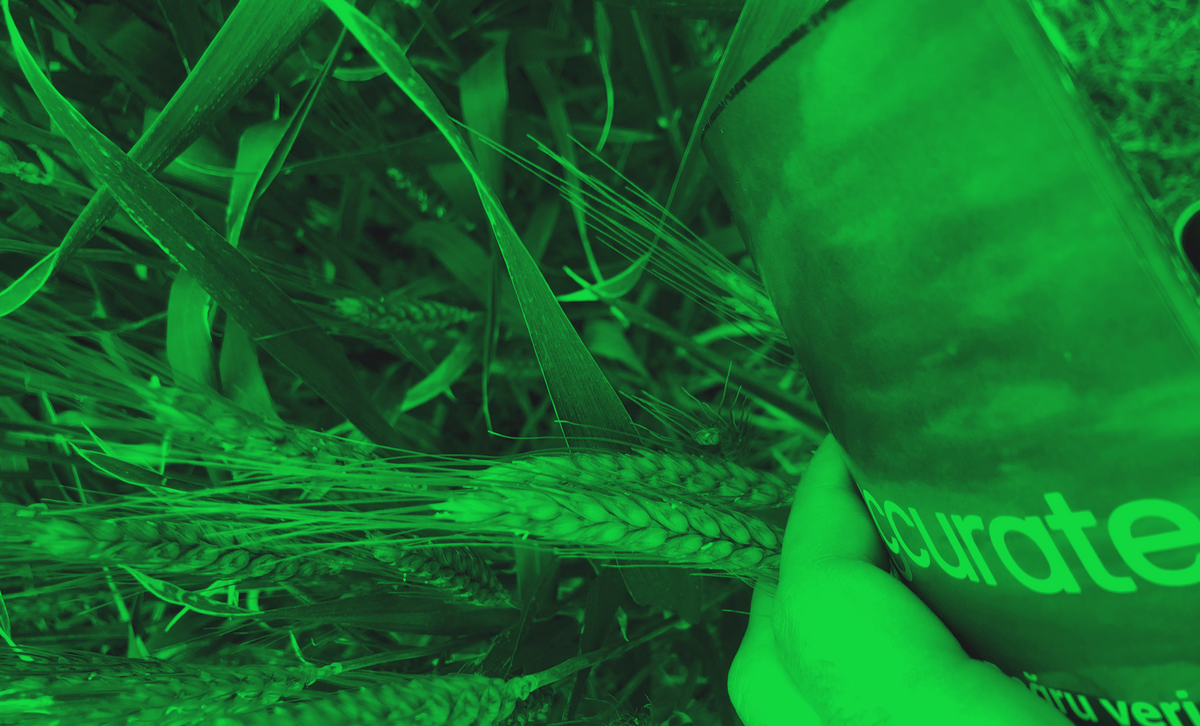
The short answer is: It is accurate. Not 100%, not 99%, but accurate enough to have a skin in the game of making agriculture more predictable today and sustainable for generations to come.
The achieved level of accuracy is more than enough to sustain existing real-world applications and enables many new ones which were not feasible, applicable or even understandable before.
Despite the fact that the differences in utilization of rural agricultural data require different methods of machine learning and imagery analysis to gather that very data, the measured accuracy in a chosen application has the same impact across almost all demands.
To give an example of our accuracy, we can discuss the following case: Field-validation of our oilseed rape classification results in all major regions of Turkey at randomly selected farms have shown that the field level precision was 96%. In terms of the value of this data and how our customers use it, we can give the following example: showing this data for 2 consecutive years would show the macro trend in a region, which will help them to strategize their marketing efforts. You can also read our blog post on detecting corn farmlands here and canola farmlands over there.
I will give more details on our approach in a bit but before that, I'd like to form a context on the question of "Why Agcurate Exists?".
Why Agcurate Exists?
It should be a blog post on its own, as it has several profound answers deeply rooted in our company culture since our foundation, but I'll give you a brief. Agcurate exists because of transparency, timeliness, and effectiveness in operational decision-making in the agri-industry. Or the lack of them.
Agcurate exists because a cotton farmer in Mali, whose product is the building block of the global textile industry, cultivates this very product at a significant personal risk - a risk that would cost him and his family almost their entire family savings. A risk exists because they don't have access to modern insurance schemes, since when they apply for insurance they are evaluated based on the socio-economic factors of developed countries (i.e. credit cards, credit scores, etc.), which are outside of their reach in the first place.
Instead, they should be evaluated on the success in cultivating their lands, thus, on their agronomy expertise. We exist because we understand the pains of 400 Million family farmers worldwide, who are uninsured and unbanked on financing and go under major risks every agricultural season. Only 3% of the farmers are insured in Sub-Saharan Africa and 22% in South/Southeast Asia. Within these regions plus Latin America, there is a $170 billion of “unmet financial demand” or “unbanked farmer demand for financing”.
Agcurate also exists because a family of four would buy a kilogram of cornflour for a price much higher than they did two weeks prior, not knowing that almost all of the companies and government branches who are the fundamental links in the global supply chain cannot forecast their operations accurately because of lacking rural agricultural data.
All of these symptoms and the problems they indicate are the main challenges in the face of creating sustainable agriculture. According to FAO, sustainable agriculture is the way to ensure answering the needs of humanity today without compromising the tomorrow of future generations. Developing sustainable food systems contributes to the sustainability of the human population.
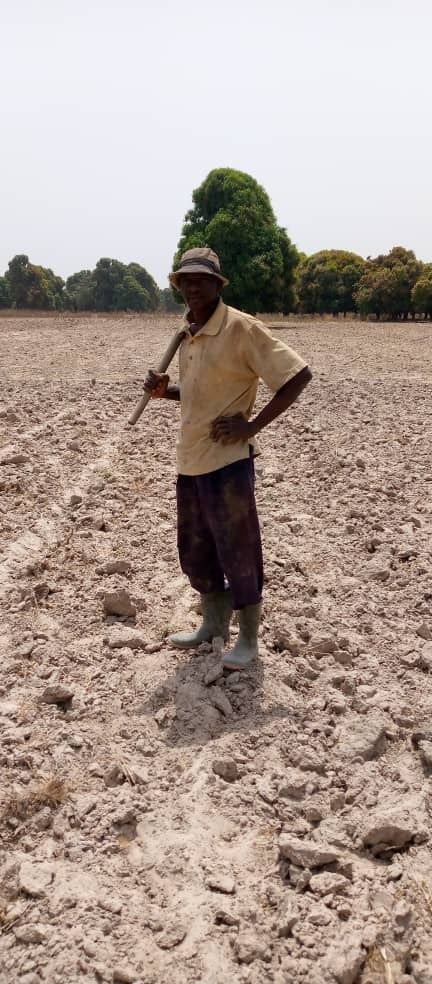
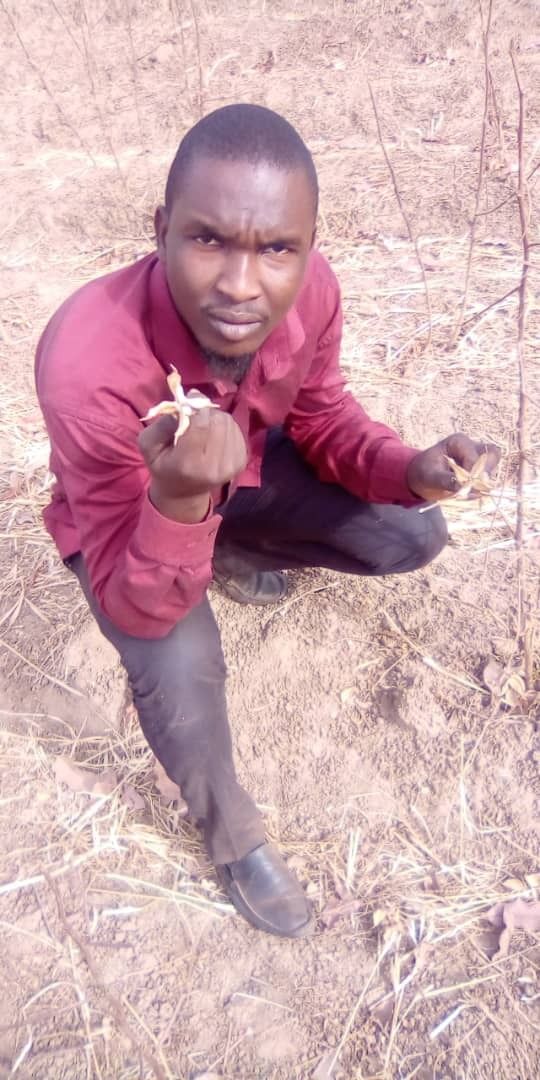
To overcome these problems, and to have a hand in creating a sustainable future, we know that the formula is simple; an agribusiness or a government office accurately knowing what happens in the field affects the monthly budget of a family of four dearly. Farmers and agribusinesses have a sense of the past of the farmlands. But that’s not enough. If they'd know what is happening right now, they could make predictions for what will happen toward the end of the season; they can broadcast their findings to other actors in the chain, and, as a result, nobody would be caught off-guard facing unforeseen, unpredictable challenges. Hence, we work every day to help develop sustainable supply chains so that we can have an impact on sustaining humanity's tomorrow.
How Do We Solve These?
We provide rural agricultural insights to interested parties - i.e. food buyers, seed providers, commodity traders, fertilizer and pesticide providers - who'll use these insights to better understand what's happening on the field and to design their next plans of action. We do this so that the decision-making process of these vital actors would not suffer from the lack of quantitative analytics.
To provide these data, we analyze satellite images periodically, and with the power of our proprietary AI we identify the crop cultivated areas. We start publishing our findings at the start of the season. After every two weeks, with new satellite images coming in, we tune our algorithm to get more accuracy in identified cultivated areas, as the new images would show more grown crops.
Using satellite images is the answer to all the problems we are trying to solve. At least to a point that would empower the operational decision-making process. Although satellite imagery has its challenges, especially the ones that require continuous research and development, we have found several solutions to overcome them. I'll list them below and briefly explain how we’ve conquered these challenges.
Field Data to Train Our Algorithms
AI requires validated field data to learn from so that it would match its learnings to its findings. This field data consists of simple information about a field: "the field at this exact point in this season is a cornfield". And that's all.
The amount of points we need from the field depends on the crop, the climate of the region, and the clearness of the parcel boundaries.
Different Crops
Different crops have different growth stages, called "crop phenologies" and thus we craft our algorithms to identify these phenologies. Also, crop pigments reflect different colors at different stages of their growth. We also count this fact in our analysis.
Different Climates
Different climates also affect the period of growth stages of the same crop. The same crop would be blooming after 6 weeks of sowing in one climate, and it would take around 12 weeks to get the first flowers under a different climate.
Unclear Parcel Boundaries
In some parts of the world, the crop cultivated areas are intertwined with forests, bushlands, etc. Thus, it is harder to identify the crops sowed in those lands because the boundaries of the fields are unclear. It is easier to falsely identify other crops as the ones we are looking for - called “false negatives”. The amount of false negatives diminishes the accuracy of the algorithm for which we need to get more field data to prevent it from happening.
With all these in mind, we've still managed to get to a point in which we only require ~20-80 field data to kick start our algorithms with satisfactory accuracy. Of course, the accuracy and adaptability of the methods grow to the full potential with a larger volume of valid field data. To get the required data, we rely on procurement from third parties or generate our own sets using company resources and collaboration with partners and customers.
Generally, our partners and customers provide initial point coordinates for a crop and we handle the rest.
Bad Weather and Clouds
Any company that uses satellite images embedded into the core of their value propositions have this problem. Clouds on the images are the common evil that we all have to fight against.
During a two weeks period, we get 2-4 images per region which we analyze. If they cover around 10000 km2 of land and if two of the images have about 20% clouds then it means for the 2000 km2 of land we can only analyze two images. For this area that is covered under clouds, our accuracy gets affected for that period. As we get more images toward the end of the season accuracy increases up to final levels (~95%) which is enough for a data-backed decision to be made.
Apart from this, using a completely different approach in satellite imagery we will be able to analyze satellite images bypassing the clouds in the near future. Although it is time-consuming, requiring in-depth research to overcome this completely, we are working toward achieving a certain level of perfection in this approach too.
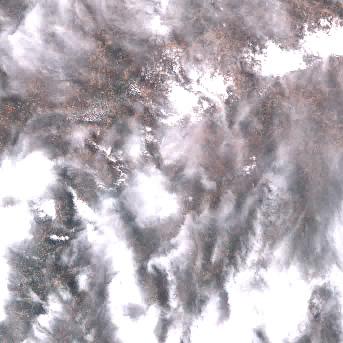
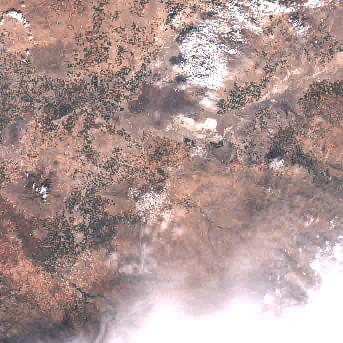
Testing The Results
How are we sure about our accuracy?
We get sample locations from our results and go check the identified fields ourselves. If it is impossible for us to go to the fields ourselves, we ask our partners to check them for us.
If we don't get enough accuracy we fine-tune our algorithms, sometimes even redesign them, we try to funnel in more validated training data and we run them again. And then we go to the fields again.
Until reaching a certain point we iterate on this process and after the first accurate analysis, we don't need to re-iterate on this very crop ever.
With the help of our partners mainly, we test our results every time we get them. But after the first successful analysis, we don't need to work on our algorithms. To this extent, by the end of 2021, we are planning to be accurate in 5 crops in 8 different countries.
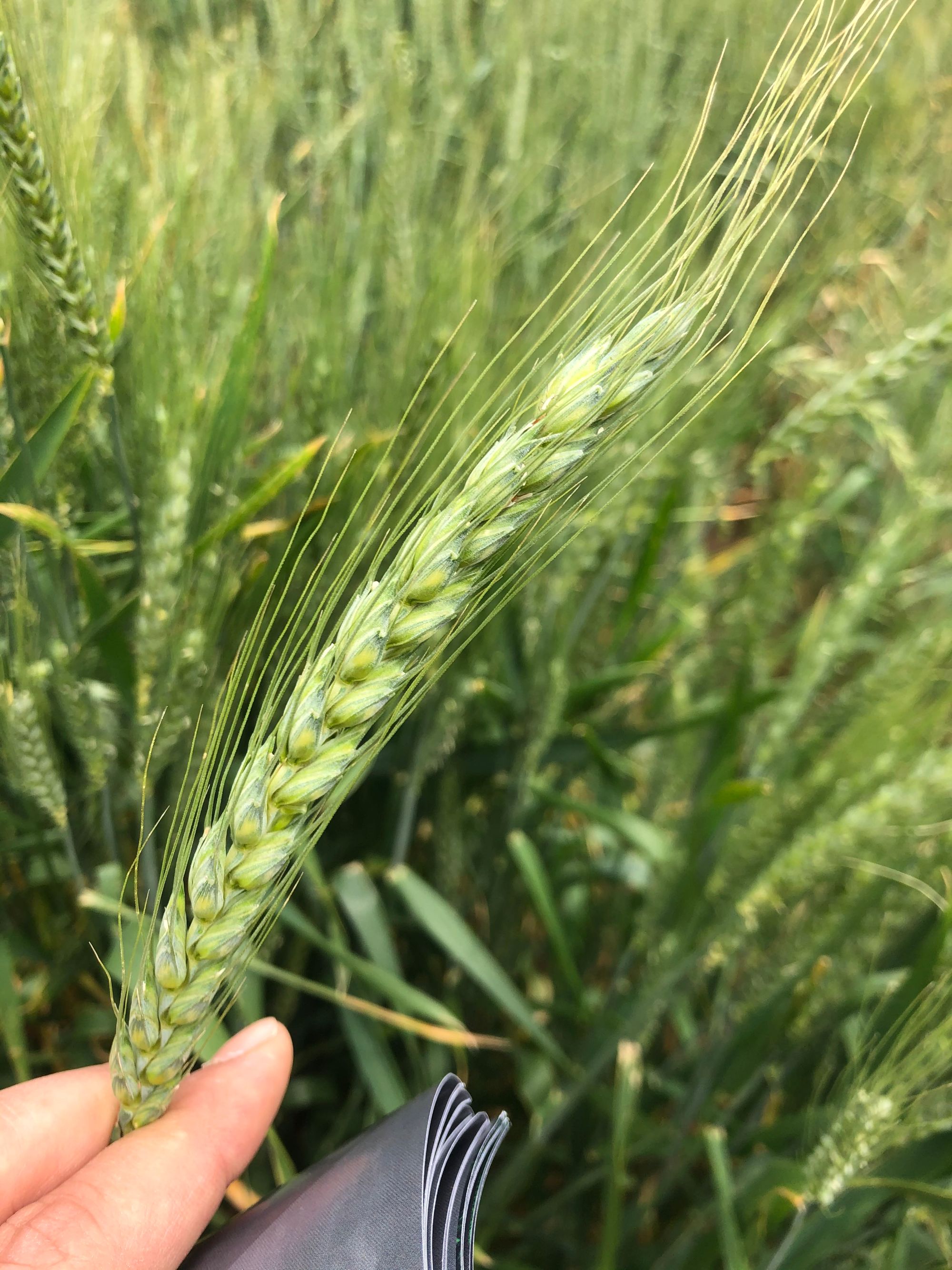
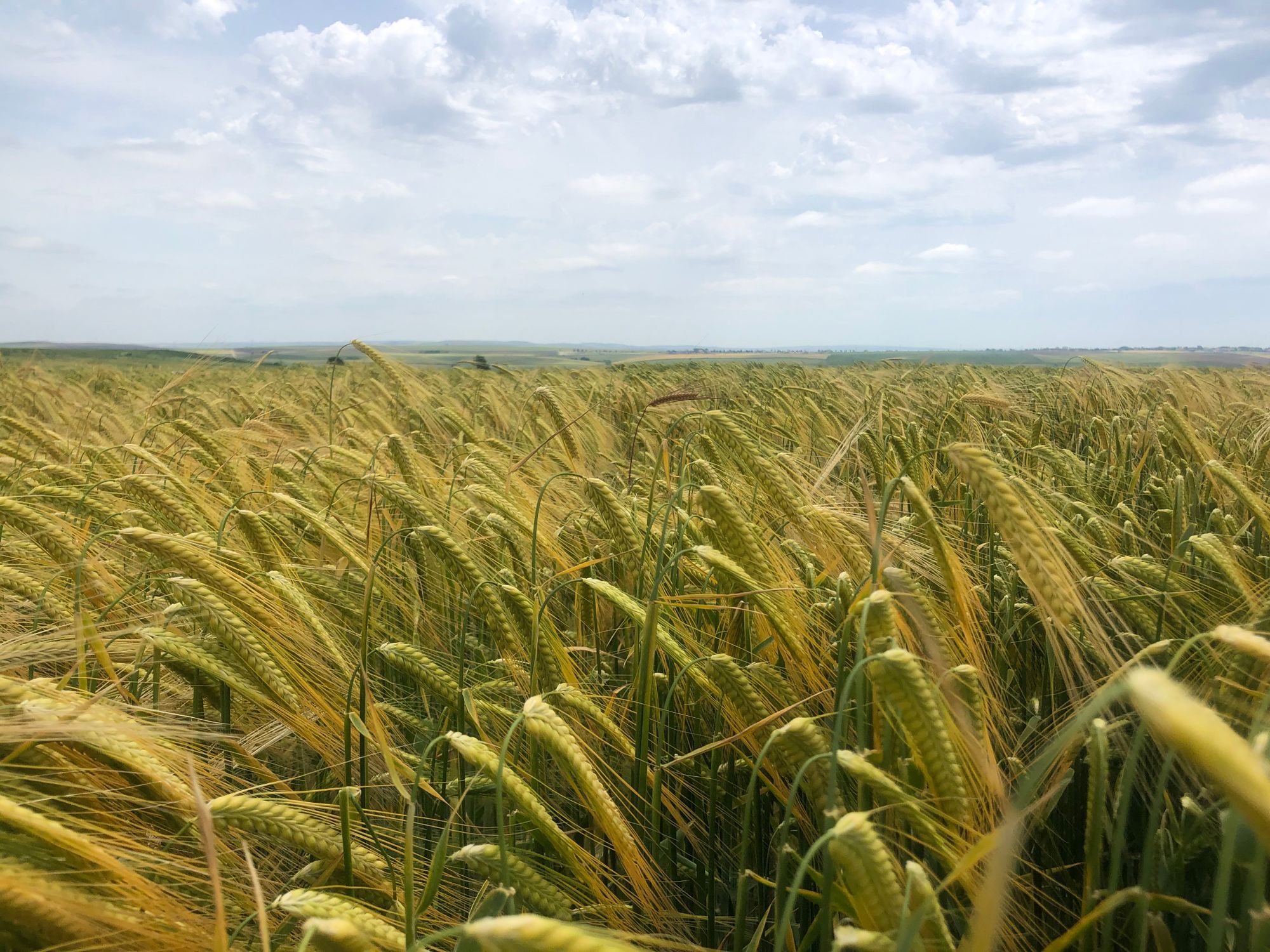
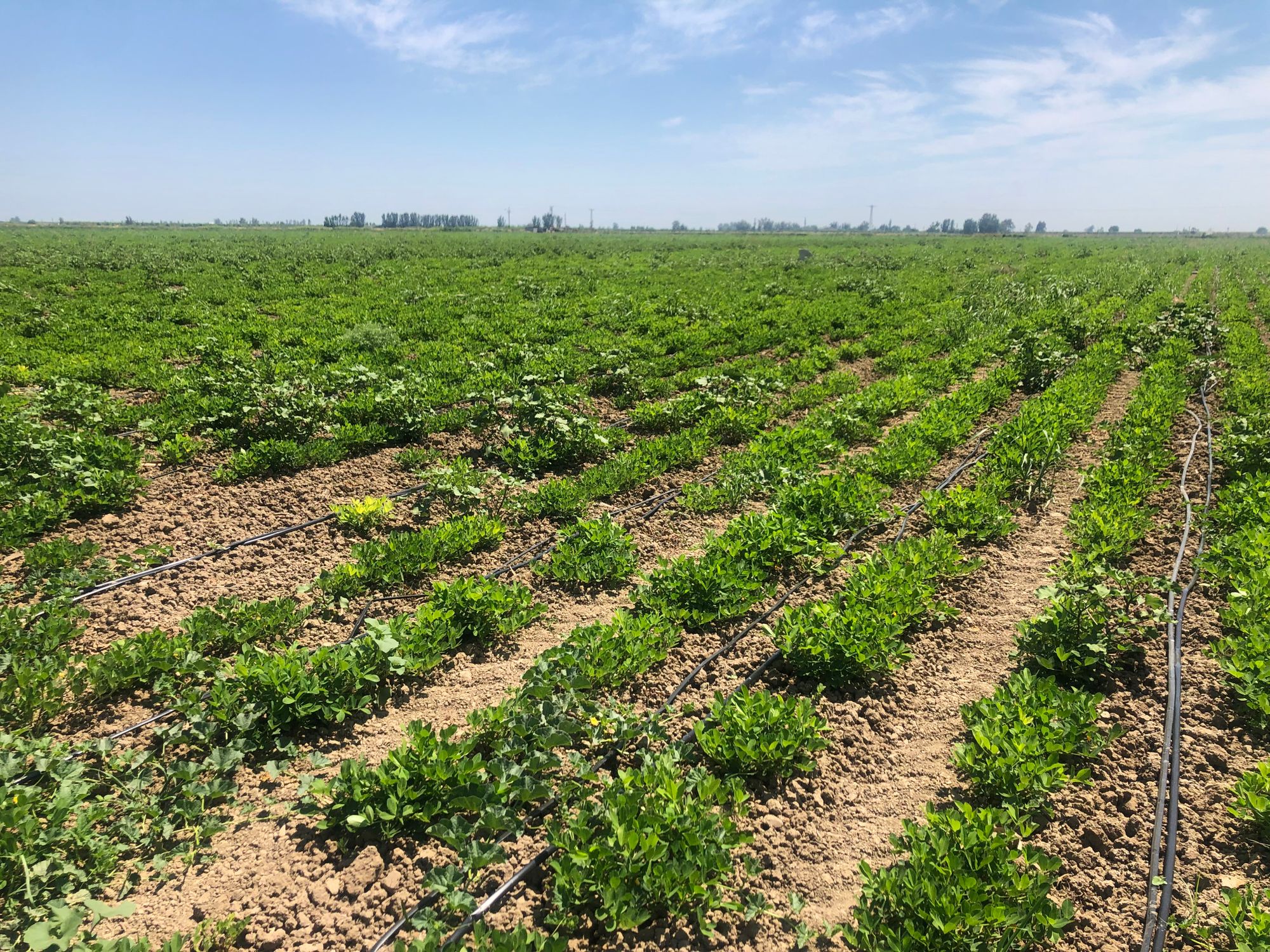
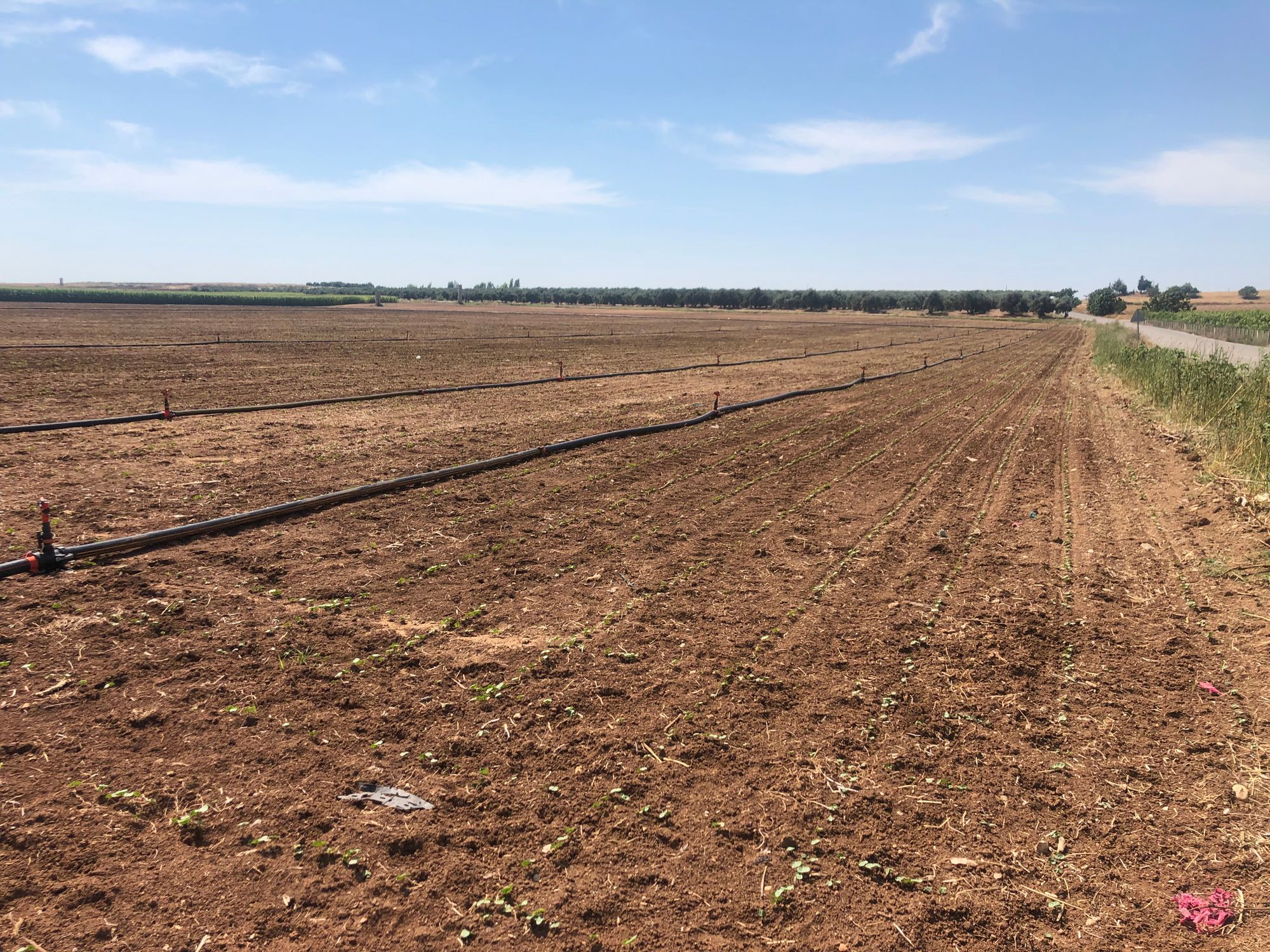
Next Steps
Testing the results is fun for us as we get more and more involved with agriculture, the fields, the smell of the lands, and the problems we are working to solve. Yet, because of the sheer amount of rural agricultural fields we need to visit globally just to test our results, this method is not scalable. As we’ve planned to identify 40 crops globally by 2024, we need a more scalable method for us to validate our findings.
Or better, for our customers to validate our findings for themselves on their own by using our platform without requiring our assistance.
As we continue to talk to our customers to understand their problems, we've decided to act on this idea and, right now, we are working on obtaining necessary tools to accomplish it. We'll announce this feature soon, after building the technical aspects of it.
Conclusion
Every single day we wake up to work on these problems and every single day we try to get to perfection. The sheer thrill and excitement of working on a problem that directly and indirectly affects billions around the world makes us cling to our cause even more.
We know for a fact that only after having a significant piece of skin in the game, getting outside of our comfort zones, accepting our boundaries, and then getting beyond them afterwards, can we solve perhaps the biggest problem in the world.